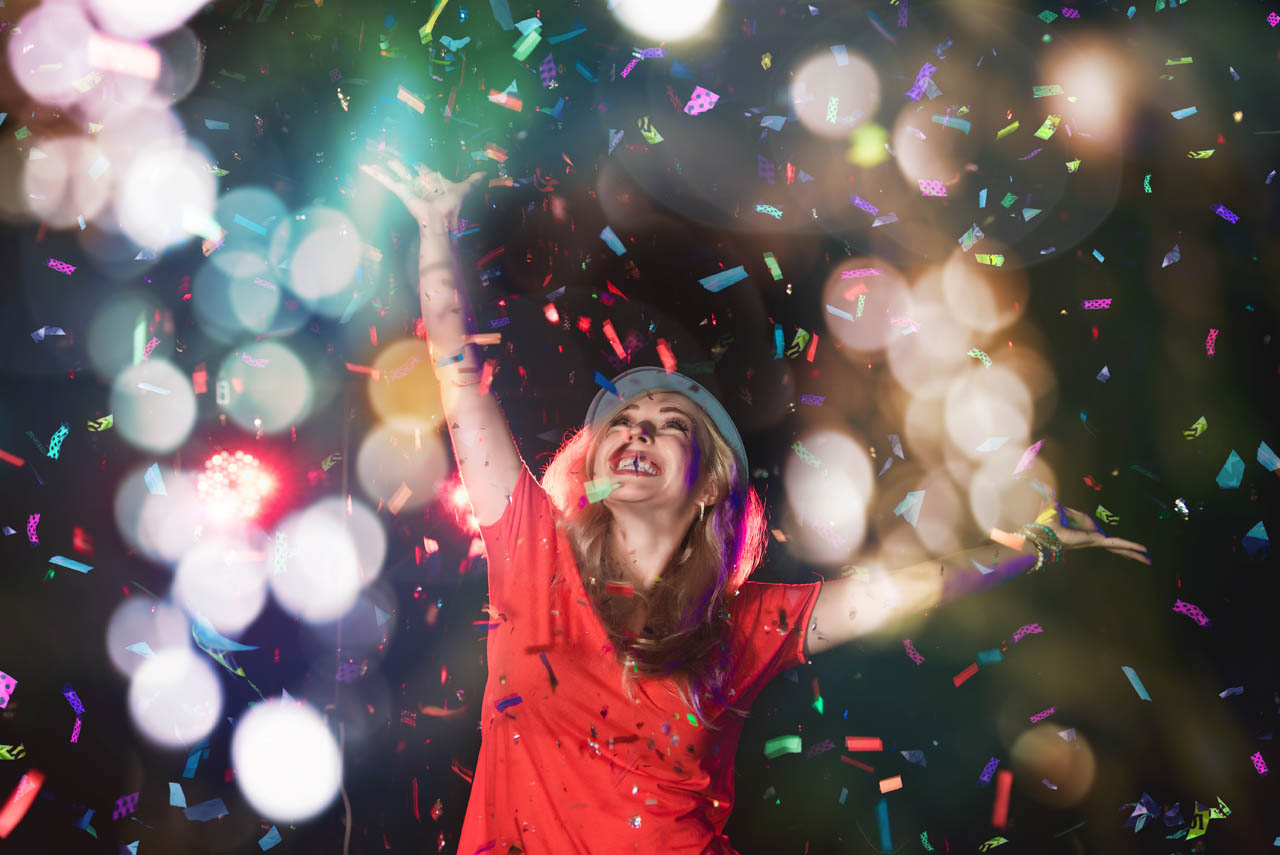
Exploring AI Reward Systems in the Era of Big Data
The exponential rise of artificial intelligence and big data has paved new ways for exploring reward mechanisms in complex systems. Modern technologies examine key parameters such as pairs and loss variance to enhance performance optimization, especially in contexts where high stakes and real-time decision-making are pivotal. This article delves into the interplay between these factors, underscoring the intricacies within AI-driven platforms and their impacts on reward balance and bonus eligibility.
Analysis of High Stakes and Bonus Eligibility in Modern Tech
In high-risk environments, such as financial algorithms or competitive gaming simulations, the integration of AI has raised questions about reward balance and the fairness afforded by bonus eligibility criteria. Researchers like Smith et al. (2021) from IEEE and the ACM Digital Library highlight that a careful calibration between risk (loss variance) and reward is crucial. This equilibrium is necessary not only for performance improvement but also for ensuring systems maintain safeplay norms, which are increasingly essential in avoiding systemic risks.
Impact of Loss Variance and Pair Dynamics on Reward Balance
The incorporation of pair-based analysis in AI models allows for the understanding of relational data dynamics, which in turn informs strategic decision-making processes. Variabilities in loss variance can both challenge and enhance a system's robustness. Utilizing high stakes and reward balance measures, modern systems can adjust parameters automatically to achieve optimal performance, balancing risk and reward efficiently. Such approaches are instrumental in sectors like automated trading, cybersecurity, and quantum computing.
FAQ: Frequently Asked Questions
Q1: How does AI manage reward balance in high stakes environments?
A1: AI systems dynamically adjust reward structures by monitoring risk factors like loss variance and pair dynamics to maintain fairness and performance.
Q2: What role does bonus eligibility play in modern tech?
A2: Bonus eligibility ensures that rewards are distributed based on performance metrics while upholding standards of safeplay and equitable risk management.
Q3: How is big data integrated into these AI systems?
A3: Big data fuels these AI models, providing a vast spectrum of information that aids in the fine-tuning of reward systems, ultimately driving greater innovations in technology.
In summary, the integrated dynamics of pairs, loss variance, high stakes, reward balance, bonus eligibility, and safeplay form the backbone of current advances in artificial intelligence and big data. Continuous research from prominent institutions underscores the necessity for balanced and ethical approaches in modern technology implementations.
Interactive Questions:
1. Which aspect of AI reward systems intrigues you the most? (pairs, loss variance, or bonus eligibility?)
2. Do you believe current bonus eligibility models sufficiently promote safeplay?
3. How would you prioritize these parameters to enhance AI performance?
Comments
AliceWonder
This article brilliantly bridges theoretical research and practical applications in AI. The FAQ section was particularly insightful!
技术达人
非常详细和深刻的分析,让我对现代科技在风险管理中的应用有了全新的认识。
DataGuru
The use of authoritative references like IEEE really strengthens the credibility of this piece. Great read!
未来探索者
文章不仅技术性强,而且观点新颖,非常期待未来更多这样的高质量内容!