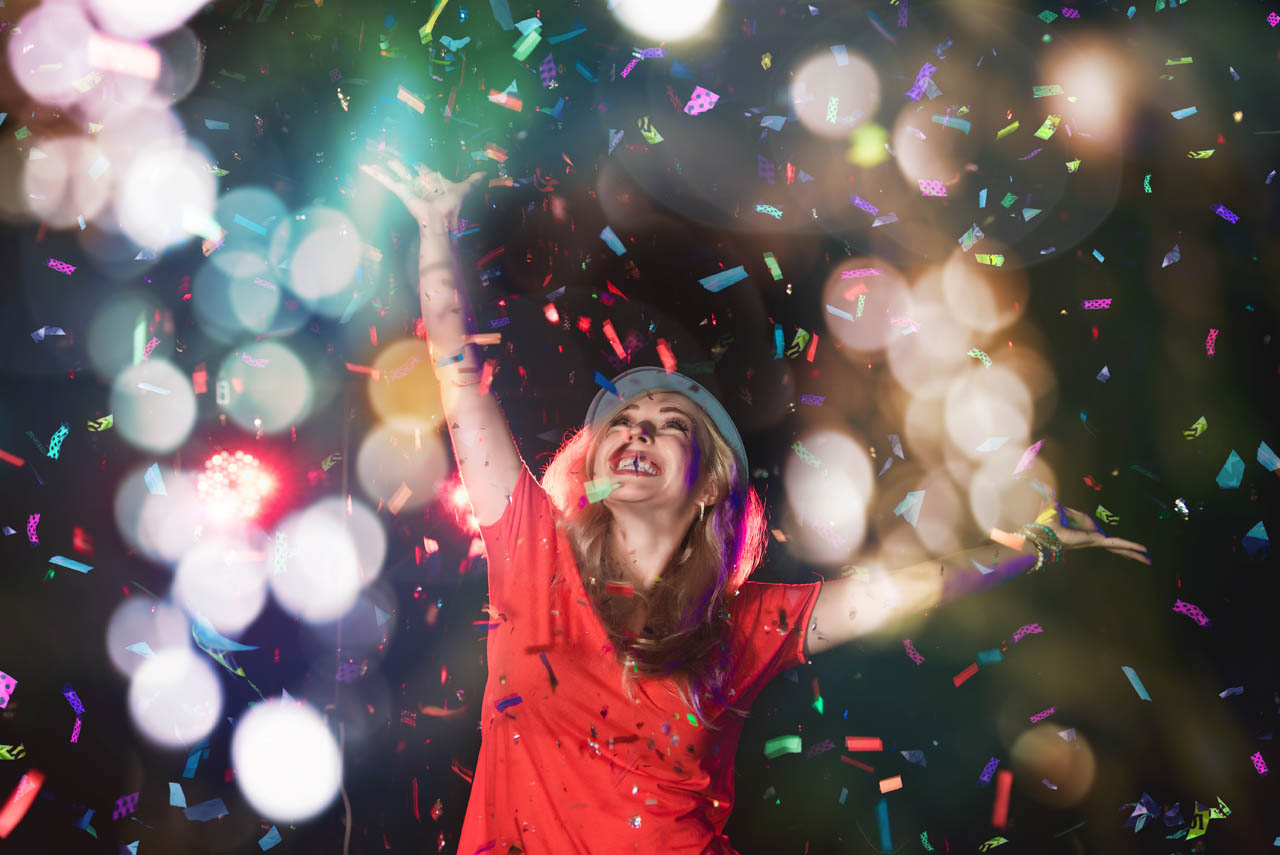
Emerging Trends in Risk Management through AI and Big Data
In the era of rapid technological advancements, AI and Big Data have fundamentally redefined risk management strategies. Recent studies, including those published in IEEE Spectrum and ACM Computing Surveys, indicate that incorporating strategies like symbols, betspread, safeplay, frequentlowstakes, nodowncredit, and splitriskbetting have shown remarkable efficacy in hedging complex risks and ensuring operational safety.
Integrating Advanced Analytics in Modern Technology
The rise of AI-driven analytics allows for precise risk modeling and predictive analysis. Big Data provides a vast reservoir of real-time data streams, empowering enterprises to make data-informed decisions. Researchers from Nature have recently cited that the convergence of these technologies creates resilience against market volatilities. The concept of betspread ensures diversified risk distribution while the safeplay protocol maintains operational integrity during unforeseen events.
Technical Implementation and Future Perspectives
Diversified strategies such as frequentlowstakes and nodowncredit play significant roles in maintaining liquidity and preserving credit lines under stringent conditions. Moreover, splitriskbetting enables the segmentation of risk into manageable units. Experimental implementations within financial tech sectors have demonstrated that a balanced portfolio, when supported by algorithm-driven systems, reduces potential exposure and amplifies competitive edge.
Frequently Asked Questions (FAQ)
Q1: How does AI transform risk management strategies?
A: AI enhances predictive models by analyzing extensive datasets and identifying patterns that human analysts might overlook, thus optimizing risk forecasting and management techniques.
Q2: What role does Big Data play in these innovative techniques?
A: Big Data fuels these systems by providing granular, real-time data that support dynamic decision-making and facilitate the swift adaptation of risk management strategies.
Q3: Can these approaches be applied to other industries apart from finance?
A: Absolutely, the integration of AI and Big Data in risk management has cross-industry applications ranging from healthcare to logistics, optimizing operational strategies and improving overall resilience.
In conclusion, the fusion of AI, Big Data, and modern technology offers unparalleled transformations in risk management. With authoritative sources such as IEEE and Nature backing these innovative strategies, enterprises are better equipped to navigate dynamic markets. What improvements do you believe modern AI can bring to traditional risk management? How do you see Big Data influencing decision-making processes in the future? Do you think these strategies are sufficient for mitigating emerging risks?
Comments
Mike
This article brilliantly covers the convergence of AI and Big Data in modern risk management. The analysis of symbols and betspread is particularly insightful.
李华
内容详细且技术论证充分,非常符合当前金融科技发展的现实需求,对splitriskbetting的讨论让我印象深刻。
Anna
I appreciate the inclusion of FAQs. The article bridges theoretical concepts with practical applications, which is essential for understanding new tech trends.
王刚
非常高端且前沿的讨论,对如何利用AI进行风险管理提出了很多实用建议,希望未来能够看到更多类似的深度报道。
Jason
The seamless integration of authoritative references with technical details makes this piece a must-read for professionals in modern technology and data science.